iNSIGHTS
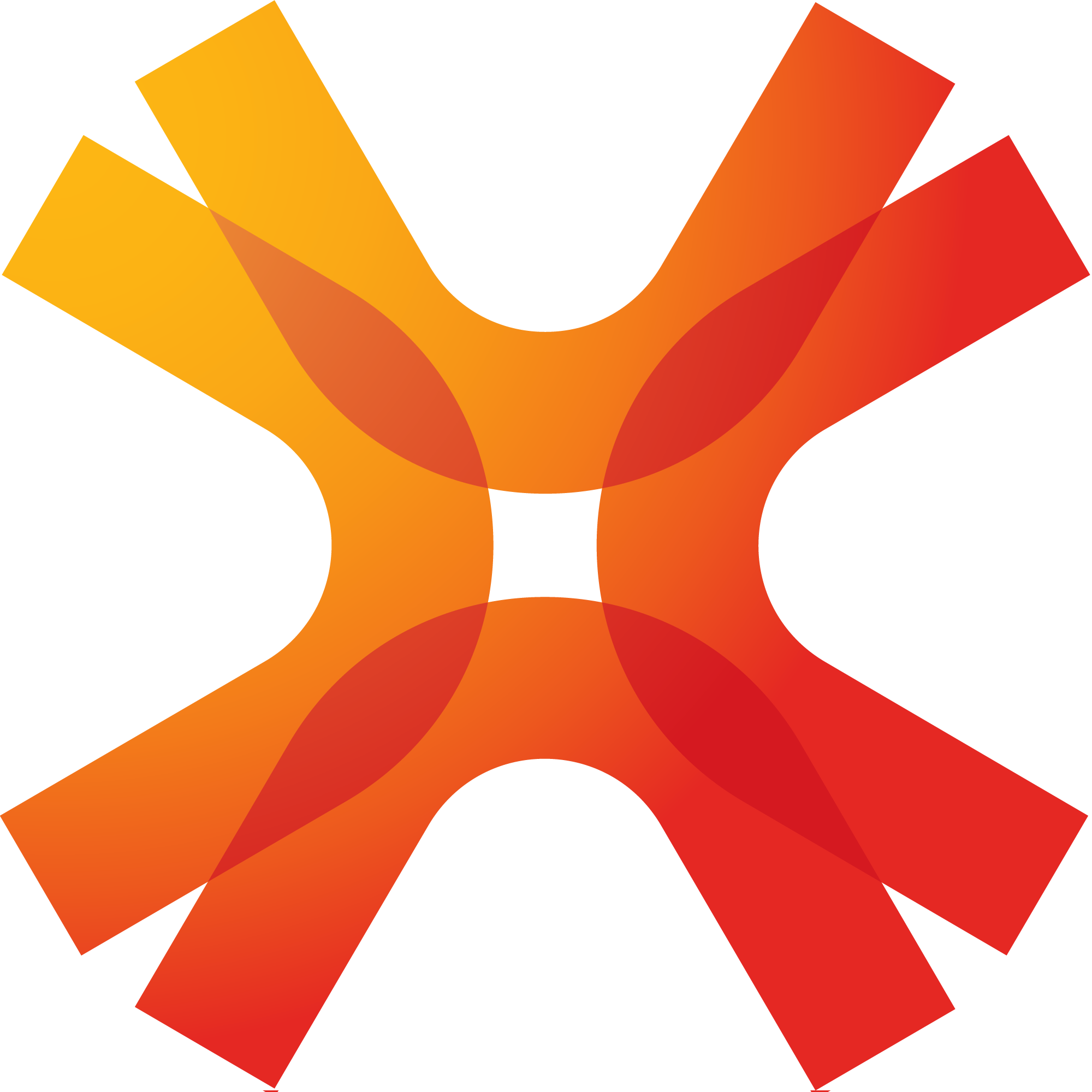
SensOre iNSIGHTS
The technical series communicating the journey from data to discovery through SensOre’s ML technology.
iNSIGHTS 1.0
SensOre iNSIGHTS on Geological Prospectivity Modelling
Transforming Embedded Geological Information into Model-Ready Data
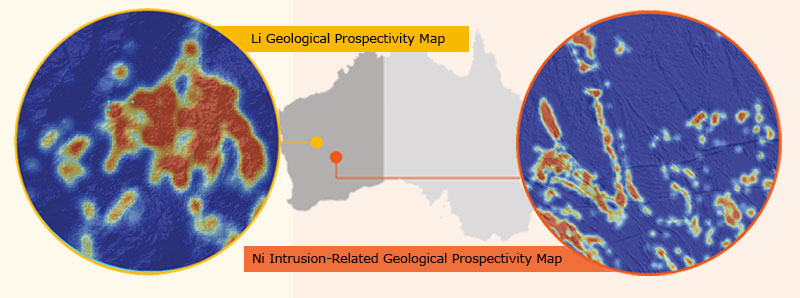
SensOre recognizes the potential of non-numeric data to be transformed to inform machine learned prospectivity models. We’ve developed advanced programmable text-mining solutions to convert geological data into numbers. Machine learning algorithms are then used to predict spatial adjacency for commodities and deposit types of interest for each point object. Knowledge extraction is driven by contiguity associations, determining the spatial relationships of specific words and phrases from SensOre’s proprietary and numerically coded geological dictionary with Au, Cu, Li, or Ni deposits. This reflects a cause-and-effect relationship between mapped geological objects and mineralisation and helps to fully utilise our geological knowledge and understanding of mineral systems of interest.
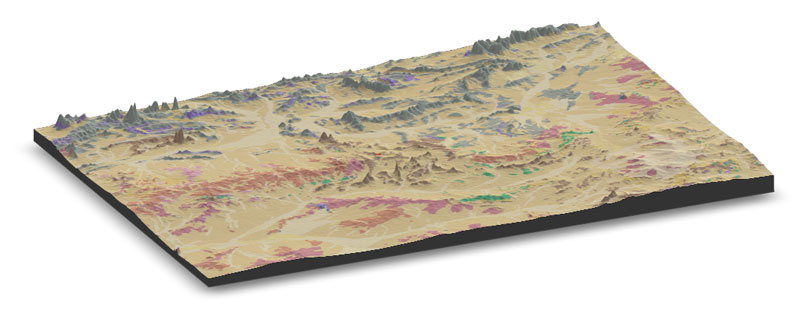
With statistical output visualisation, SensOre has constructed new geological prospectivity maps over WA for a range of commodities and deposit types including Cu-VMS, LCT Pegmatite, Ni Intrusion-Related, and Phosphate. SensOre’s geological prospectivity models are on track to be extended to other states and continental Australia as the SensOre data cube expansion evolves towards completion over the coming months.
SensOre’s insights into geological prospectivity can be used in exploration for terrane and region selection, and for project generation and ranking. Strategically, these maps can be used to probabilistically assess the geological prospectivity of any tenement for the commodities modelled, making them an invaluable tool for business development decision-making.
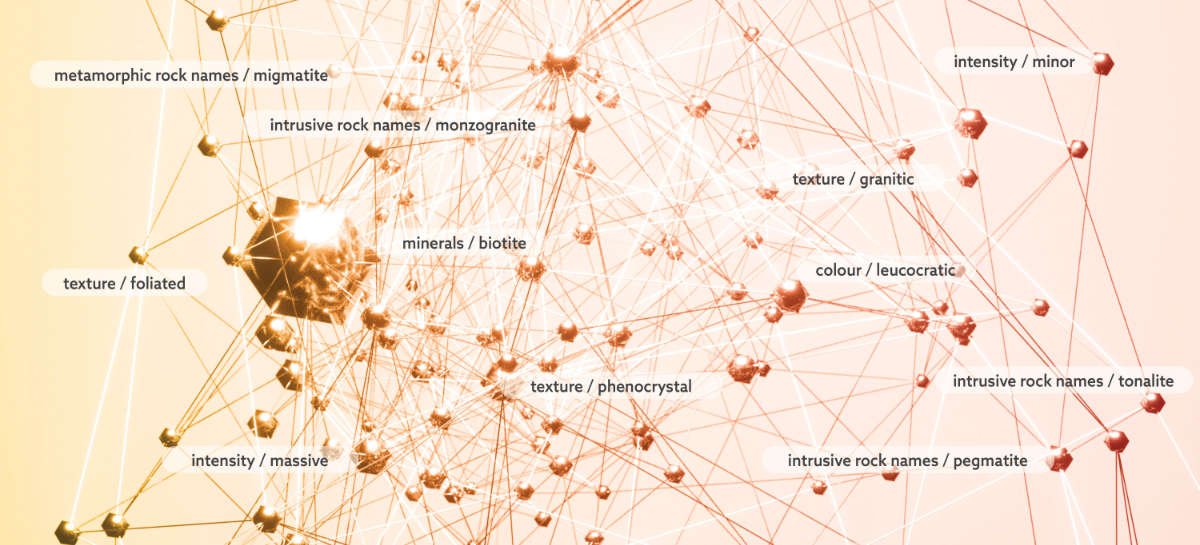
SensOre’s advanced programmable text mining solutions.
iNSIGHTS
How do you get access to these resources?
Contact SensOre to maximize your exploration success by utilising our propriety advanced machine learning capabilities.
iNSIGHTS 2.0
SensOre iNSIGHTS on Growing Australia Data Hypercube
Significant State-and Continent-Scale Expansion of Data Capture
SensOre Ltd is boldly changing the way exploration targeting is carried out by harnessing and fusing the vast quantities of underutilised geoscience and environmental data from the public domain into its Australian Data hypercube. SensOre’s most valuable (non-human) asset is its Data Hypercube. The data in the database resides in a common, globally scalable gridded geospatial architecture consisting of layers of geoscience data including geology, geochemistry, and geophysics. It is central to all SensOre models generated on open data and underpins our targeting services allowing SensOre to generate high-quality, data-driven exploration targets.
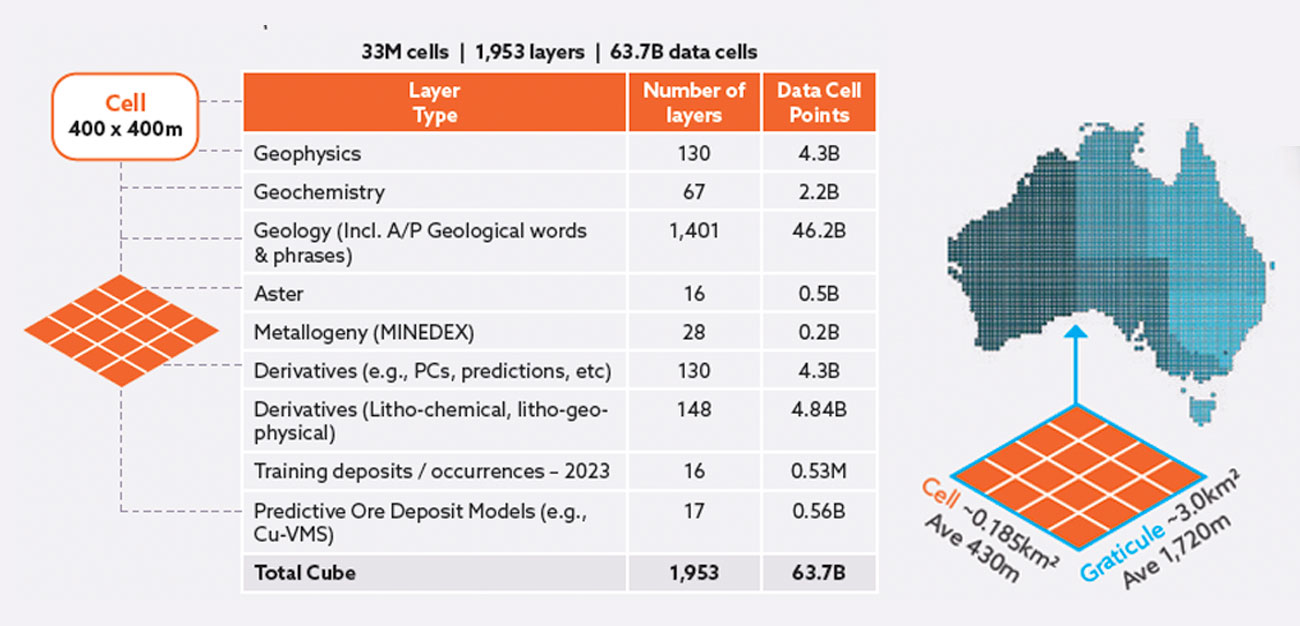
State-scale geoscience data is now ingested for WA, SA, and NSW
SensOre achieved a significant milestone of compiling, cleaning, merging, and ingesting the vast quantities of all openly available state-scale geoscience data across SA and NSW, adding to the completed data hypercube for WA. This was accomplished with the support of industry clients in SA and competitive government co-funding in NSW from the Critical Minerals and high-Tech Metals Activation fund. The Data Hypercube currently contains approximately 2,000 layers of geoscience data with more than 63 billion data points occupying the cells within the Data Hypercube.
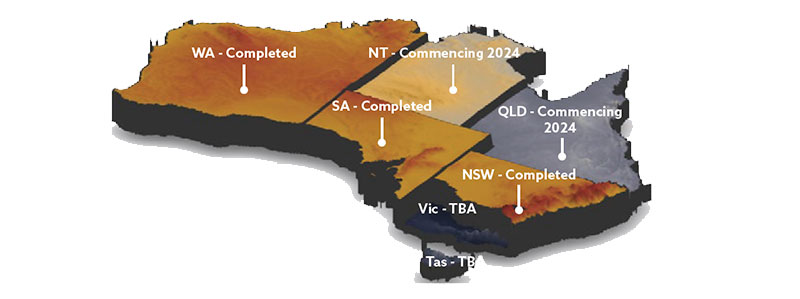
The Data Hypercube includes SensOre’s Discoveries Database, an extensive metallogenic database of compiled and cleaned deposit and mineral occurrence data from many sources. This database is updated quarterly and is expanding to include more commodities. This database provides accurate point location, resource footprints, and mineral endowments and grades calculated from (mines, resource, reserves, and historical production), deposit depth including tested deposit depth and depth to top of deposit, mineral deposit type. The Discoveries Database is one of our most valuable data assets as it functions as one of our primary training datasets containing 61,660 occurrences for gold, nickel, copper, and lithium.
Combined, the Data Hypercube and the Discovery Database are deployed in SensOre’s machine learning (ML) workflows to target critical minerals, including Au, Ni, Cu, Co, PGE’s, Li and REE’s.
Continental-scale geoscience data expansion
A second significant milestone was achieved this year with respect to our continental-scale geoscience data compilations. We have made considerable advancements in acquiring, processing, compiling, and merging continental-scale geophysical and geochemical data. SensOre has ingested CSIRO’s ASTER, and hydrogeochemistry data.
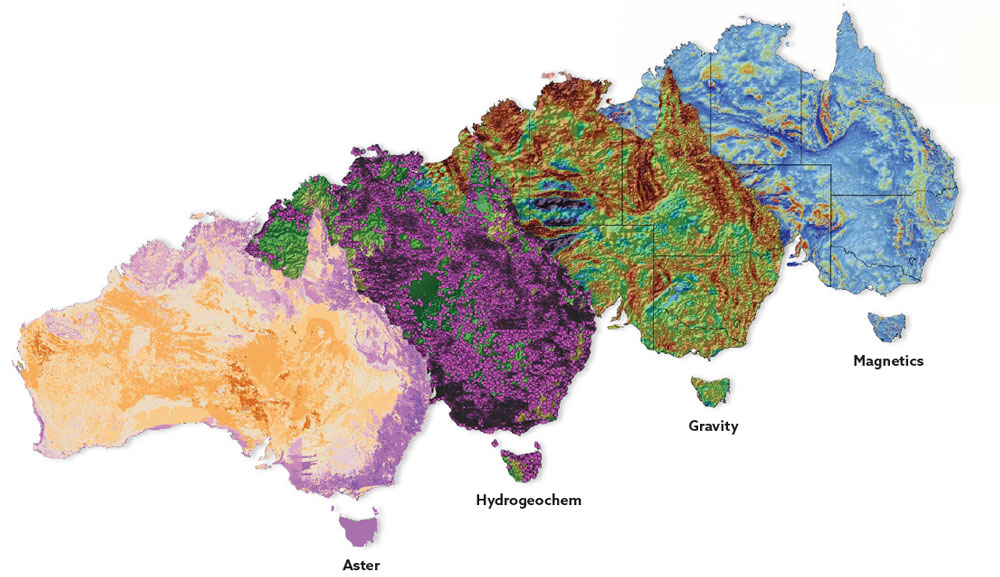
Intrepid Geophysics has delivered their advanced processed continental merged compilations for magnetics, gravity and radiometrics data and approximately 54 derivative layers each for magnetics and gravity and 27 derivative layers for radiometrics. These data sets were added to our 430 m resolution Data Hypercube over the continent and are now suitable for application of machine learning algorithms as well as to our 80m resolution grids which aggregate to some 165 billion data cells over the continent.
Why are SensOre’s Data Hypercube expansions worth talking about?
- SensOre’s Data Hypercube expansion is addressing a protracted, industry-wide dilemma namely, the underutilisation, under-appreciation, and poor management of both new and legacy geoscience and environmental data that continues to obscure the potential utility of geoscience data.
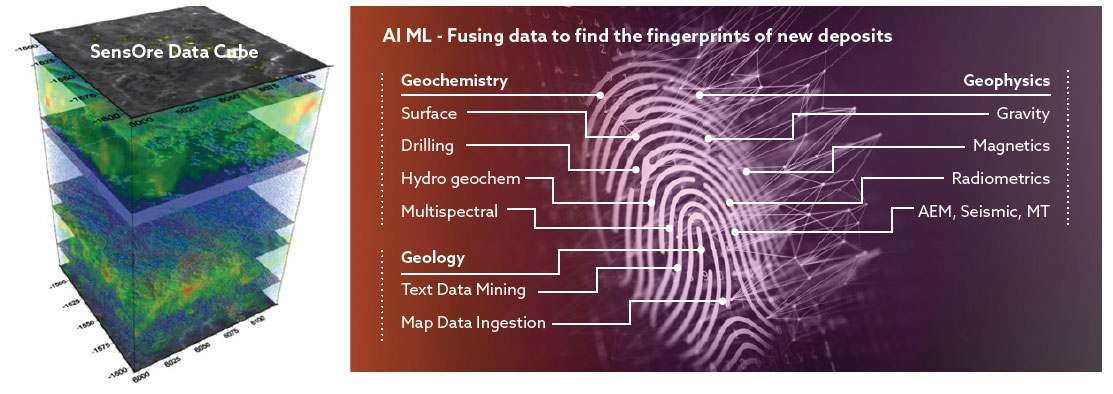
- We’ve done the hard yards of compiling, cleaning, feature engineering and fusing massive amounts of geologically and environmentally significant data into a highly curated, proprietary database so you don’t have to.
- The construction of Data Hypercube represents several millions of dollars of investment and years of human resources dedicated to curating geoscience data conservatively estimated to have cost industry more than $20B to collect across three states.
iNSIGHTS 3.0
SensOre iNSIGHTS on igROCK and Simclust ML Tools
Supervised and Unsupervised ML Tools Generate Geological Insights from Geochemistry
SensOre Ltd has productionised a range of innovative ML tools and workflows to derive geological knowledge from geochemistry data. The power of these tools are realised by leveraging data from our substantial geoscience Data Hypercube and subject matter expertise in both data science and geosciences. SensOre’s ML solutions address protracted, everyday problems and challenges faced by geologists across the mining value chain and provide information and insights that allow decisions to be made on how projects should progress. Two of our most sought-after ML tools are igROCK and SimClust for Geochemistry. When used in tandem, these supervised and unsupervised approaches produce powerful and reinforcing results that increase understanding and confidence in the data collected.
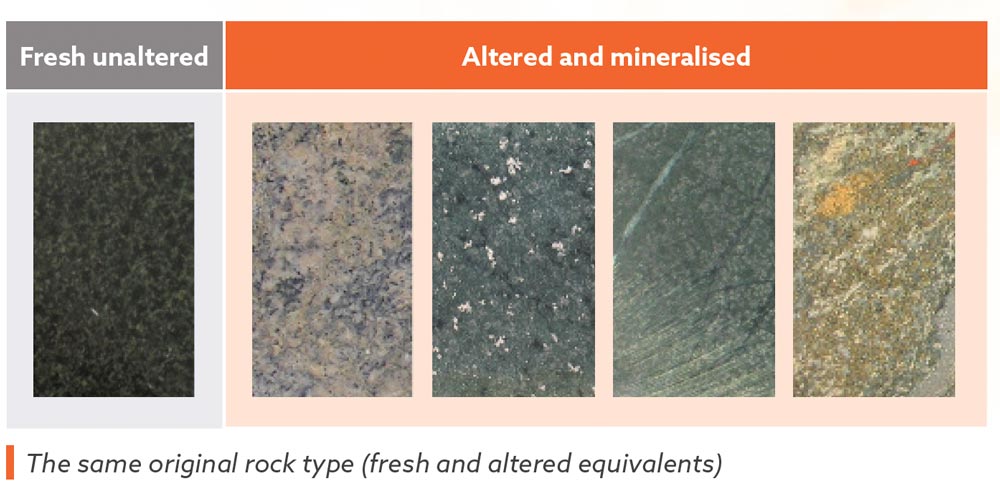
IGROCK: A supervised ML tool that leverages SensOre’s Data Cube
Correctly identifying protolith types is critical when defining the spatial distribution of geological sequences. Especially where hydrothermal alteration and/or weathering and/or metamorphism is pervasive (and let’s be honest, if the rocks are interesting rocks they will often be altered!). To make things even more difficult a massive amount of the samples geologists have to log come from RC or aircore drilling programs. To help geologists make sense of their geochemical samples SensOre has developed igROCK – a supervised igneous protolith classification system. igROCK has been trained using igneous geochemical databases comprising over 400,000 samples. It uses the extended multi-element geochemistry to automate the classification of igneous rocks into 42 different rock types. Key to igROCK’s performance is the appreciable time SensOre’s geoscientific staff members have spent cleaning (and relabelling) these training databases prior to training the igROCK model (you would be surprised how many samples originally labelled as fresh ultramafics are running less than 1 % MgO!). Protolith identification is typically subjective and experience based. igROCK provides an automated solution to help geologists spend more time on the fun stuff (like interpreting where fluids have come from and planning where to put the next hole).
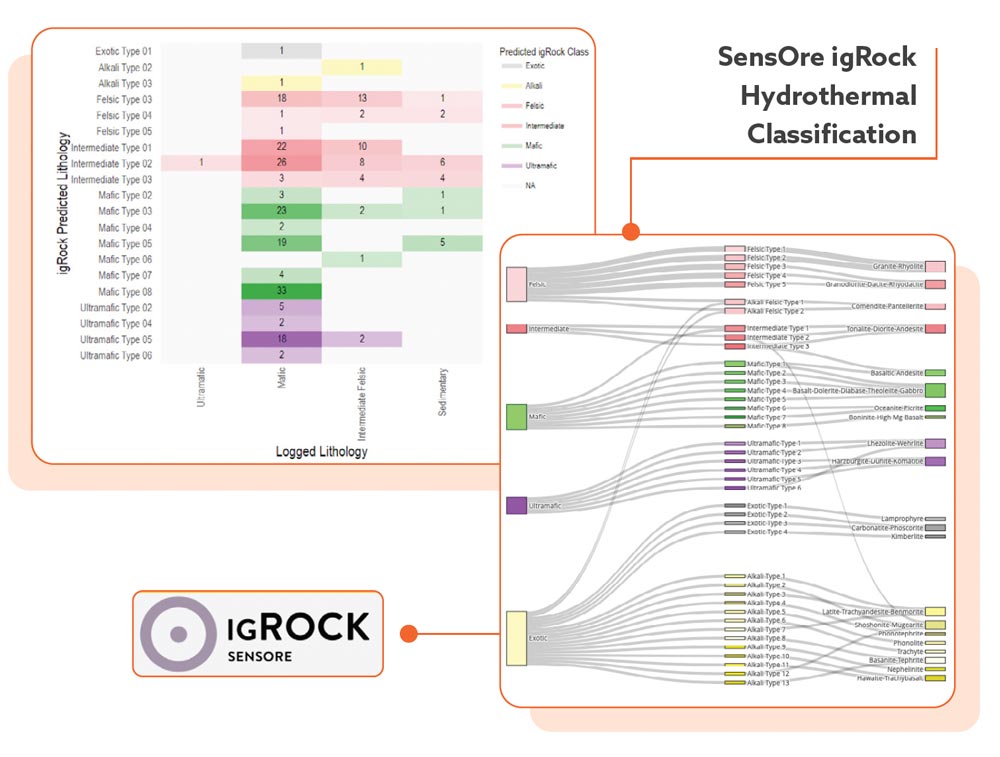
SimCLUST™️: An unsupervised ML tool that leverages SensOre’s Data Cube
SimClust Geochemistry is an unsupervised ML tool used to identify and classify natural geochemical populations of surface and drillhole point data using multiple, multivariate statistical models. The results can be used for rock characterisation, exploration vectoring, identification of altered equivalents, and differentiating regolith from saprock to name a few applications. The image below shows the Kundana Drill hole assay data in SimClust space.
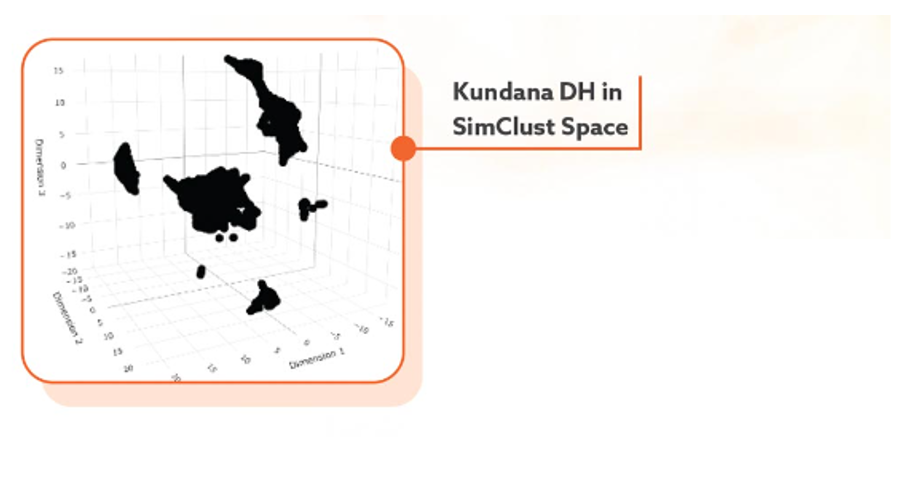
Supervised and Unsupervised ML: A match made in heaven
When the results from igROCK are used to inform the unsupervised outputs from SimClust, magical things happen. When combined, igROCK and SimClust provide the geologist with valuable insights regarding different protoliths and altered equivalents and can help reinforce or challenge previous interpretations.
The coloured image below shows the Kundana Drill hole assay data SimClust clusters trained by igROCK.
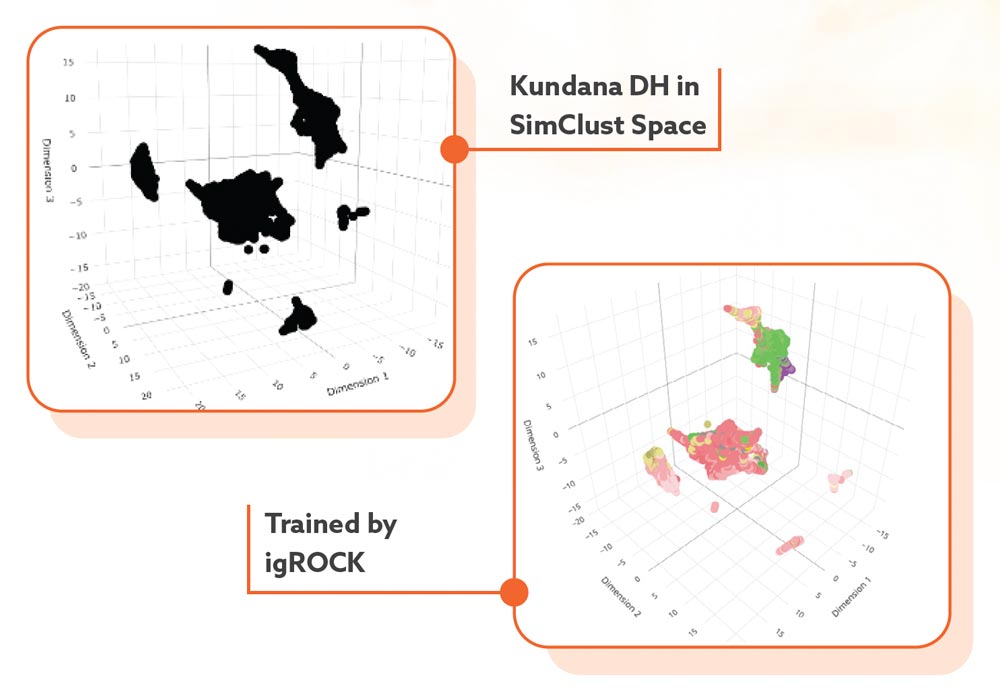
The geologist can now answer questions such as which samples within these clusters are mafic? Are there multiple mafic groups or just one? Are there multiple fractionation trends representing multiple magmatic pulses in the AOI? Does a cluster represent multiple subclusters? In our example using the Kundana Drillhole assay data, igRock is able to inform SimClust there is ultramafic, mafic and felsic units in the first cluster, there is a felsic and intermediate subcluster in the second cluster, and that the remaining clusters are all felsic.
The map below shows the igROCK results plotted spatially, revealing coherent geological trends for the ultramafic, mafic and felsic units.
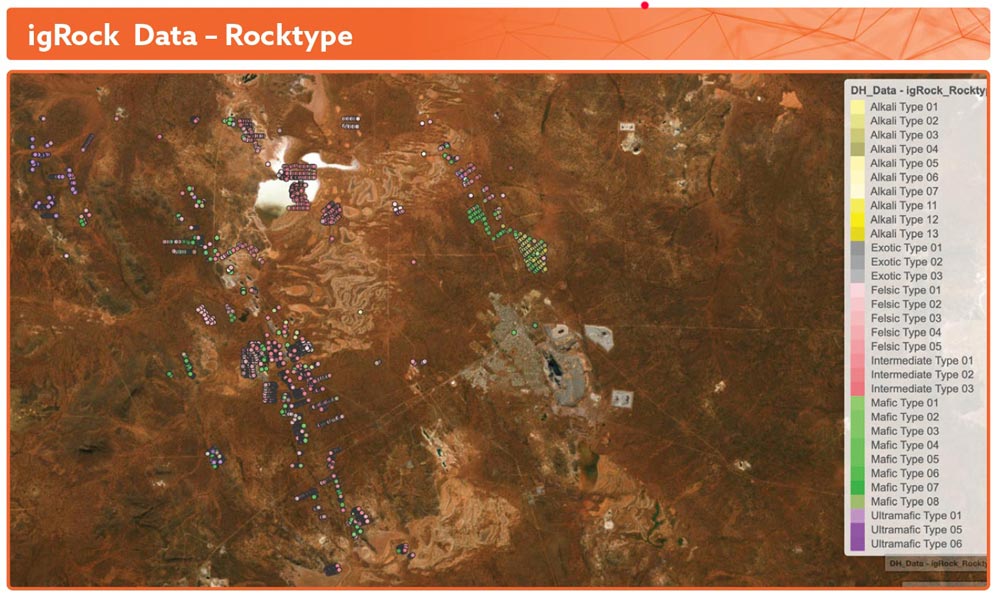
A Joint Supervised and Unsupervised ML: Case Study
The case study presented here is from bottom of hole chips collected from aircore surveys from an area near Mulga Bill. As if logging Aircore chips isn’t hard enough, the weathering at Mulga Bill is deep (150 m!), and the alteration is pervasive. The image below represents the geologists original interpretation of the samples.
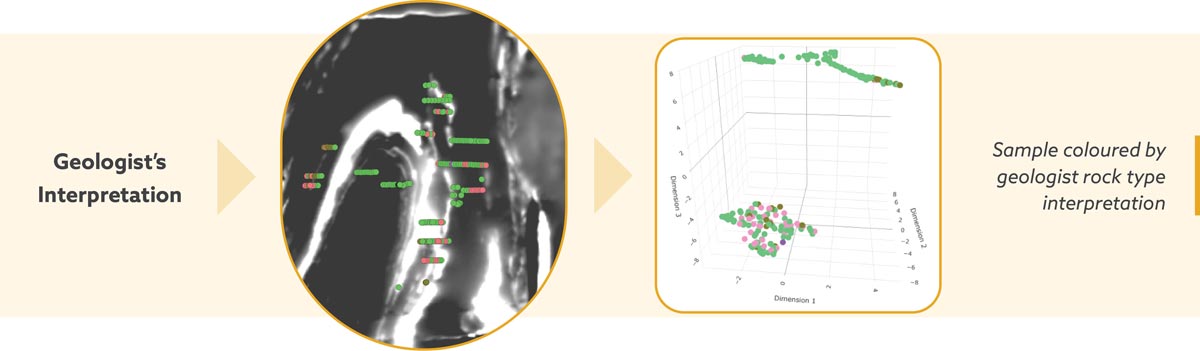
The next image shows the results from igRock. As you can see there are some significant differences between the two classifications. The original logging considers the samples in the east to be predominately mafics, while igROCK has classified the samples in east as being predominately intermediates with some lenses of ultramafics. That’s great, but how do we know igRock is providing reasonable predictions? When combined with the outputs from SimClust we can see that igROCK’s interpretation is far more consistent. igROCK is also able to inform SimClust as to the location of cluster boundaries.
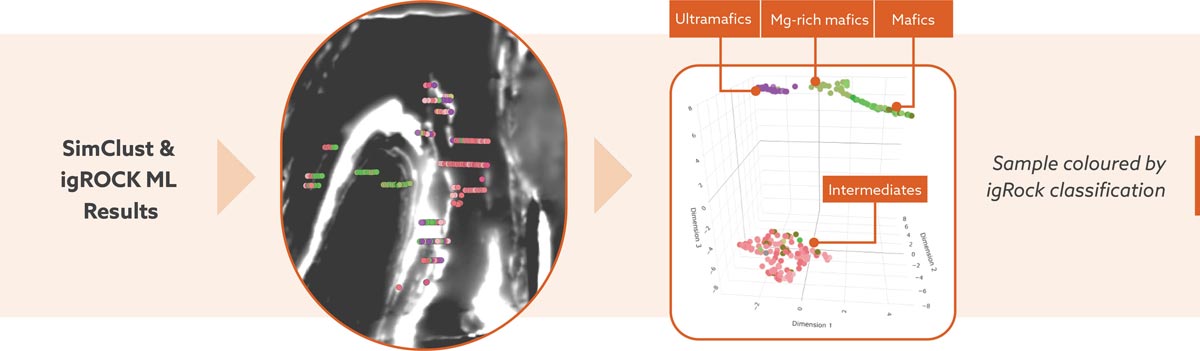
Why are SensOre’s SimClust and igROCK ML tools worth talking about?
SensOre’s SimClust and igROCK geochemistry workflows are powerful proxy mapping tools in low-data density regions and in areas under cover.
The igROCK protolith classification system provides information critical in defining the spatial distribution of geological sequences where the primary mineral assemblages are obscured or have been destroyed by hydrothermal alteration and/or weathering. igROCK also provides a data-driven approach to classify difficult to log samples from RC and air-core programs.
SimClust provides powerful unsupervised validation for igROCK as well as a number of SensOre’s other supervised ML tools including AGLADS and iGOSSAN, which can be found in our brochure.
iNSIGHTS 4.0
SensOre iNSIGHTS on Predictive Models
Changing the Exploration Landscape Through Intelligent, Data Driven Area Reduction
Previous SensOre Insights have focused on our advanced programmable text-mining solutions for extracting geological information from maps and point datasets, the expansion of our data hypercube, and our supervised and unsupervised ML tools.
In this contribution, we focus on the value-added products, outputs, and insights generated from combining our Data Hypercube, Discoveries Database and ML technology in SensOre’s Predictive Models. We share how these innovations can enable a shift from conventional exploration practices to an Industry 5.0 future, delivering next-generation target predictions through the combined power of machine learning and big geoscience data, augmented by expert human analysis for decision-making.
The Current Exploration Landscape:
The lack of innovation in exploration is often cited as a reason for declining discovery rates and rising costs per discovery using conventional methods (Table 1, Figure 1).
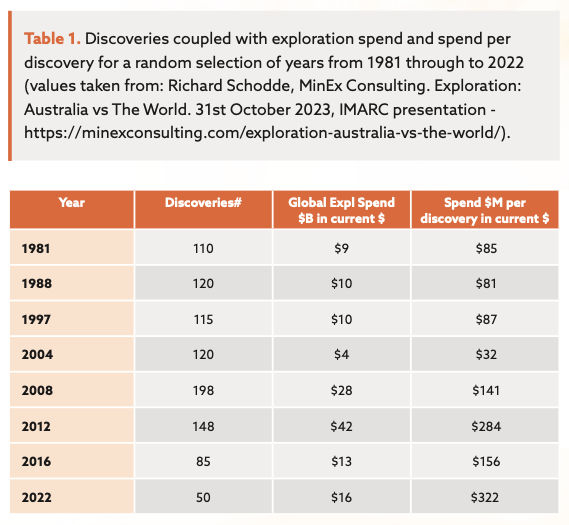
The time and cost investments per major discovery can be tracked across each stage in the exploration pipeline to highlight the current industry performance (Figure 1).
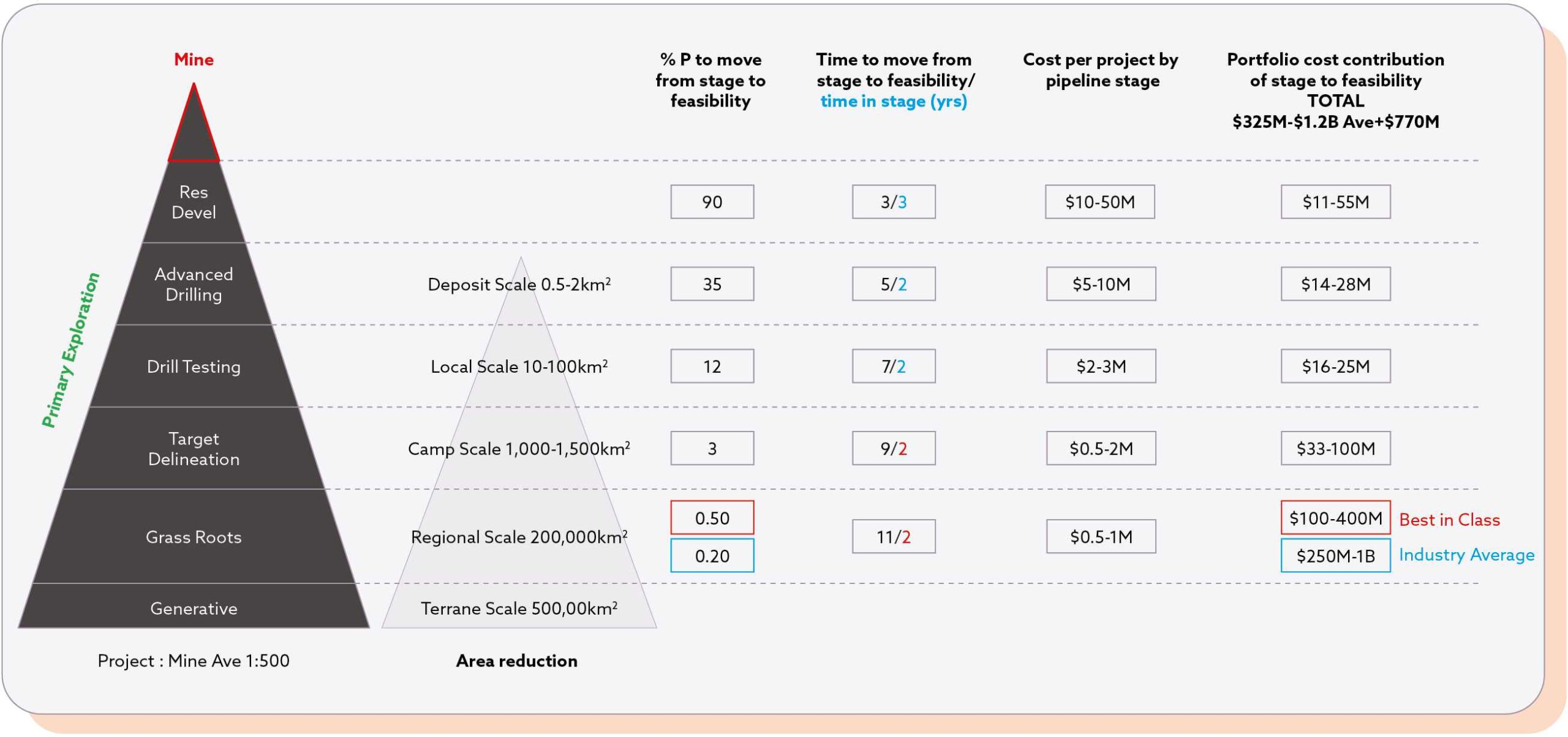

The largest gains in time and cost efficiency can be achieved by improving success rates in the earliest parts of the exploration pipeline by making better predictions on where ore deposits are located. Even a small increase in the probability that a grassroots exploration target is good enough to pass the area reduction threshold would significantly boost the number of projects reaching feasibility. While no single company can shift the dial alone, adopting new technology-based innovations across the industry can collectively generate more high-quality targets that can be efficiently tested and moved up the pipeline.
SensOre’s Innovations in Predictive Modelling Enabled by our Data Hypercube and Discoveries Database:
SensOre aims to lead the industry in improving discovery rates while reducing the cost and environmental impact of discoveries through our innovations in predictive modelling. These models identify higher quality targets using a robust data-driven ML approach. We strive to help the industry make better decisions about where to drill and where not to, using extensive geoscience data to predict the locations of future Tier One and Two mines.
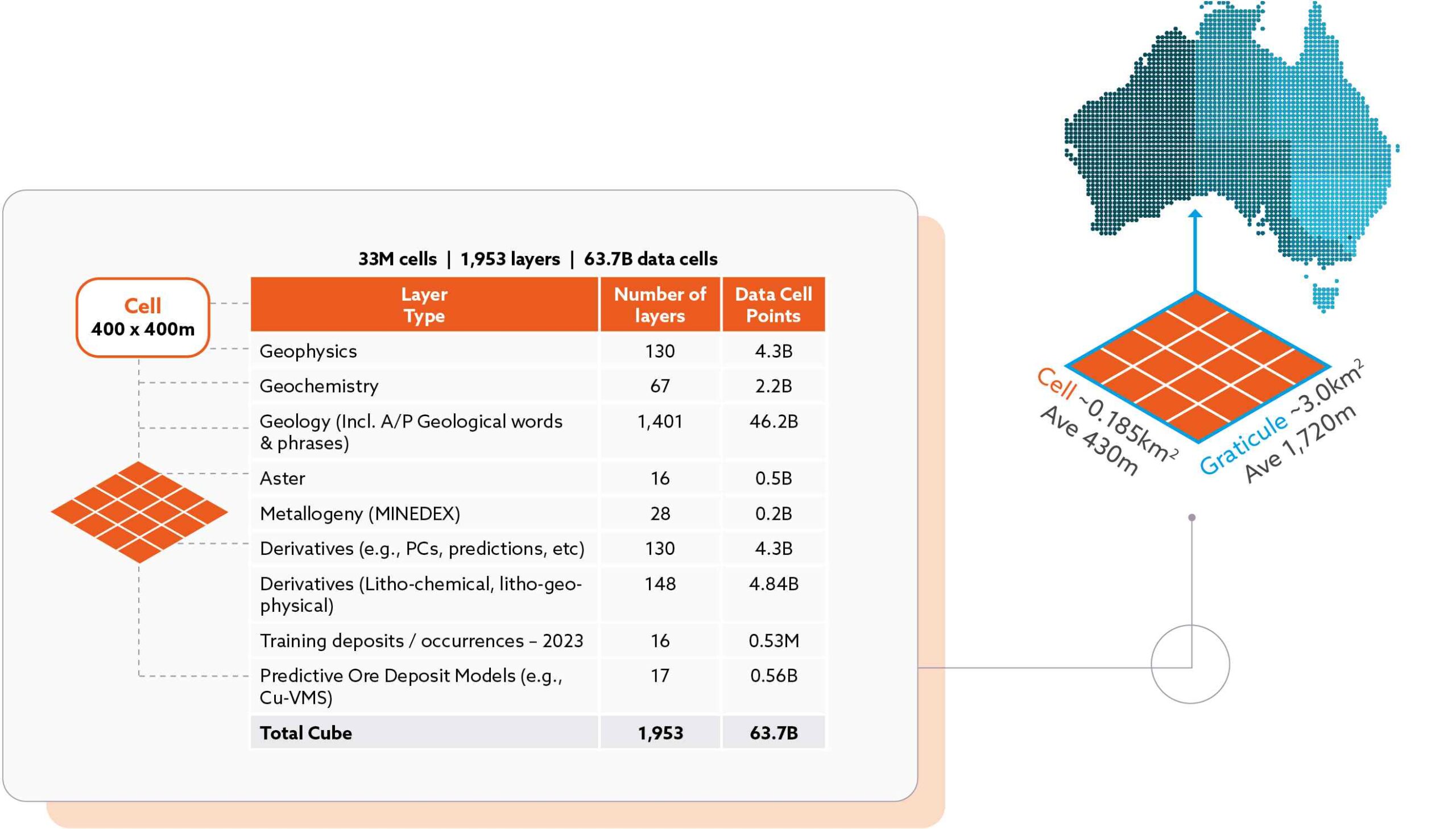

Our Predictive Models are unique because they are built on our massive, curated data hypercube, which integrates and fuses multidisciplinary geoscience data in a structured and scalable geospatial architecture (Figure 2). The data hypercube houses the largest, most accurate, and precise deposit database, serving as training and testing data for our models. This enables us to create robust models across various scales, from Terrane to Region to Camp-scale.
SensOre’s Innovations in Predictive Modelling:
SensOre’s investment in curating data to build the Data Hypercube and Discoveries Database allows our geoscientists to develop unique predictive modelling approaches for any commodity of interest (Figure 3).
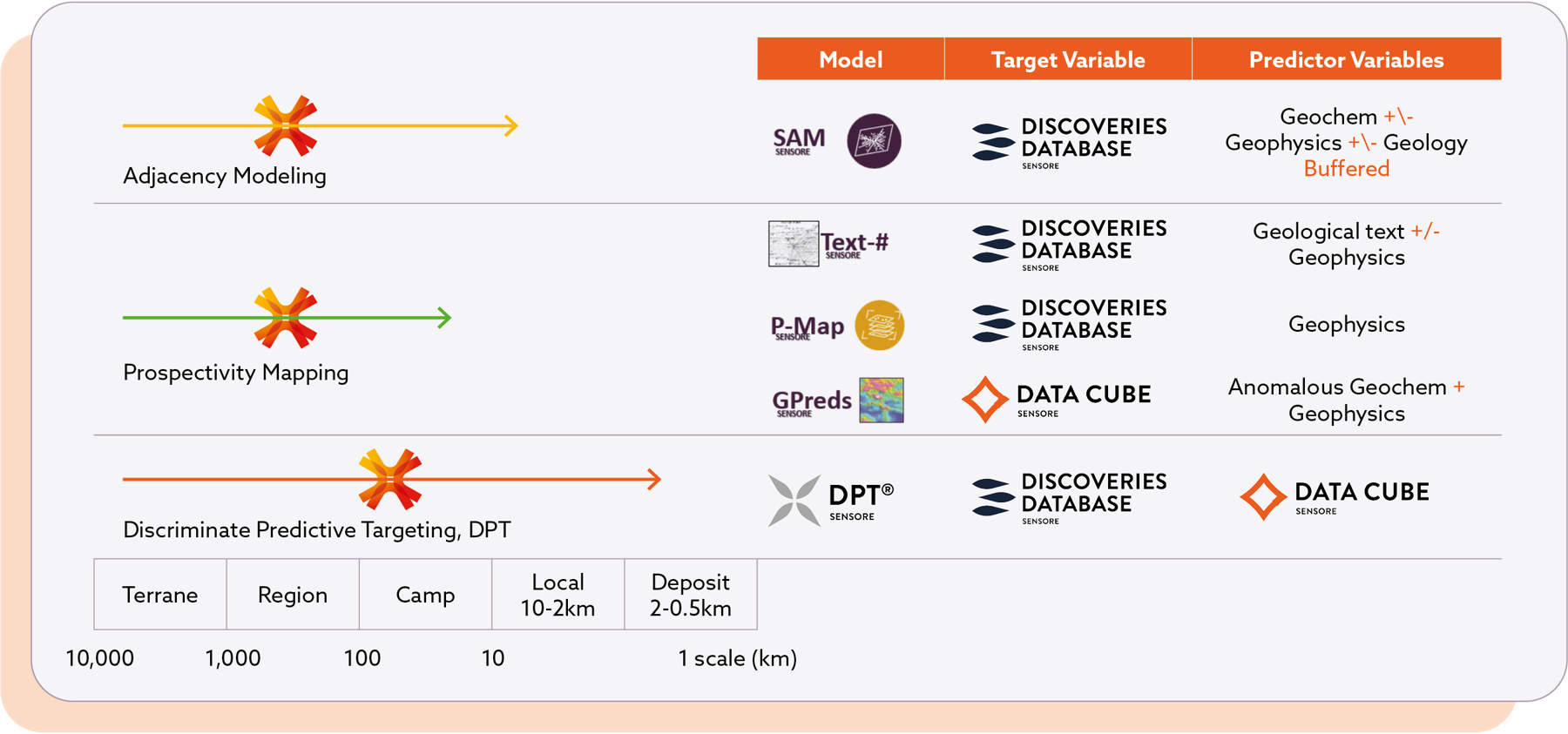

One example is our GPreds model series. The GPred modelling concept creatively fuses geophysics and geochemistry to address a significant challenge in predictive modelling of economically valuable metals: label imbalance. In Western Australia, only a few hundred, and often only a few dozen, data cells out of a state-wide population of 13 million contain significant economic endowment. By training and testing advanced geophysical derivatives using anomalous geochemistry in up to 90,000 data cells with more than 47 elements, SensOre minimizes this issue (Figure 4). SensOre’s geophysical layers, delivered by our wholly owned technology and software company, Intrepid Geophysics, support this process at all scales.
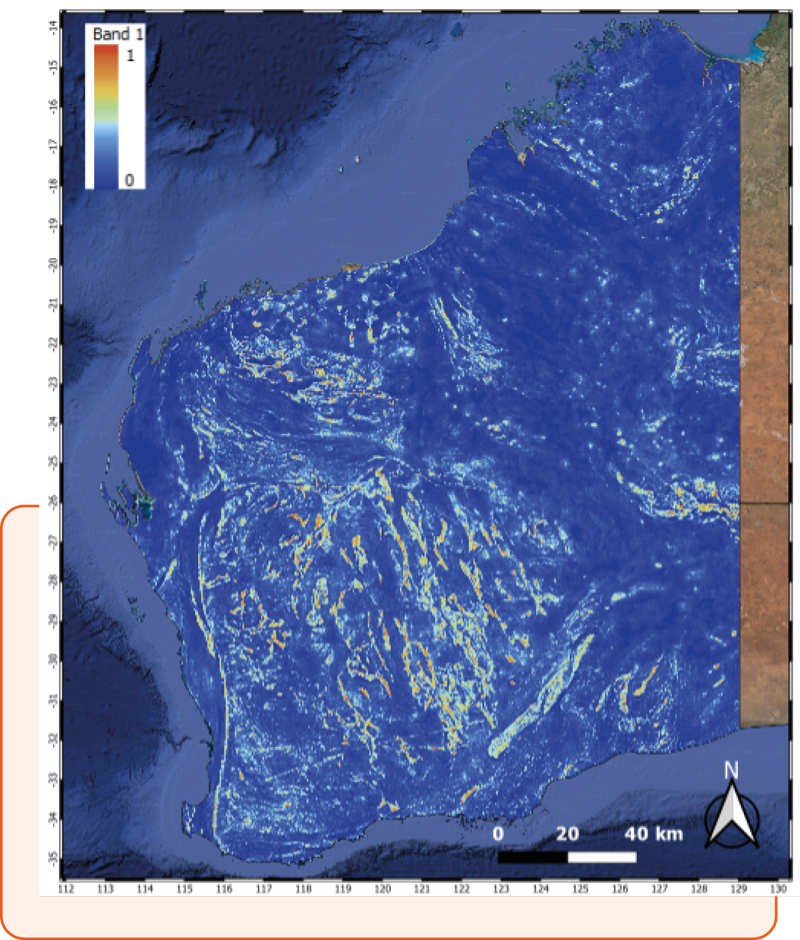
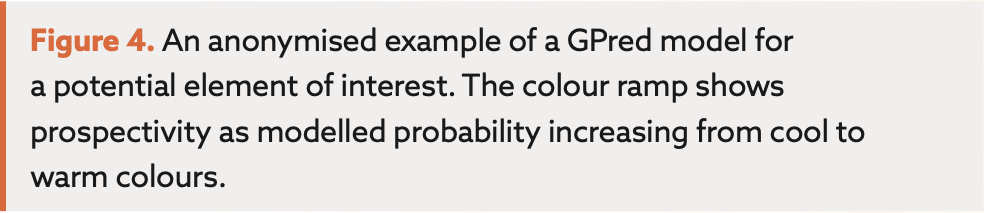
Creating Value through Innovation:
To demonstrate the value of our GPred models for Western Australia, we apply a data-driven metric to assess and rank existing tenure prospectivity for Au, Cu, Ni, and Li using probability categories. We conducted a raster zonal analysis, attributing July 2024 WA tenure with the maximum modelled GPred anomaly probability values and probability categories intersecting the tenements.
The raster zonal analysis for the GPred Au model reveals that only 7% of WA tenements are on ground predicted to have greater than a 90% chance of significant Au anomalism (Table 2). This ground is held by a few hundred companies out of over 3,000 listed holders in WA, accounting for 1,921 out of 25,807 existing exploration and mining tenements with mineral rights in WA. For Cu, Ni, and Li, only 15%, 17%, and 6% of the tenements, respectively, are on ground predicted to have greater than a 90% chance of hosting significant concentrations of these elements (Table 2).
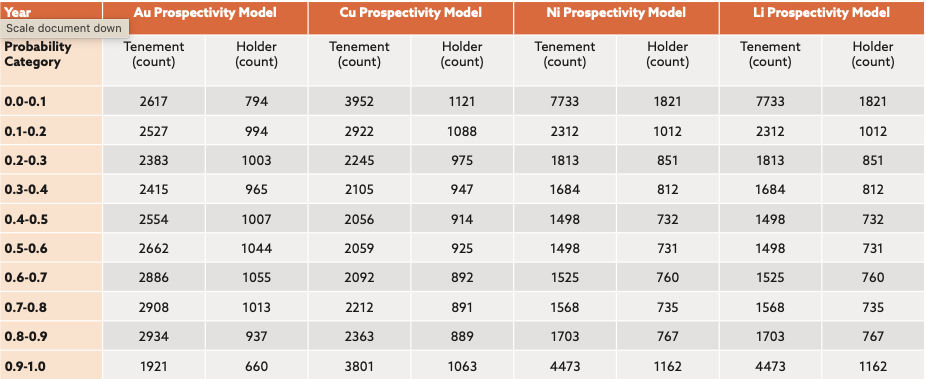

These statistics can be sobering, but there are further insights. Competition is tight even within the highest probability category. There are winners and losers, and closer inspection can yield valuable insights. For instance, for Au, among the 660 unique holders of tenements in the highest probability category, we can identify those with the largest number of tenements, suggesting the size of land holdings on the most prospective ground (Table 3).
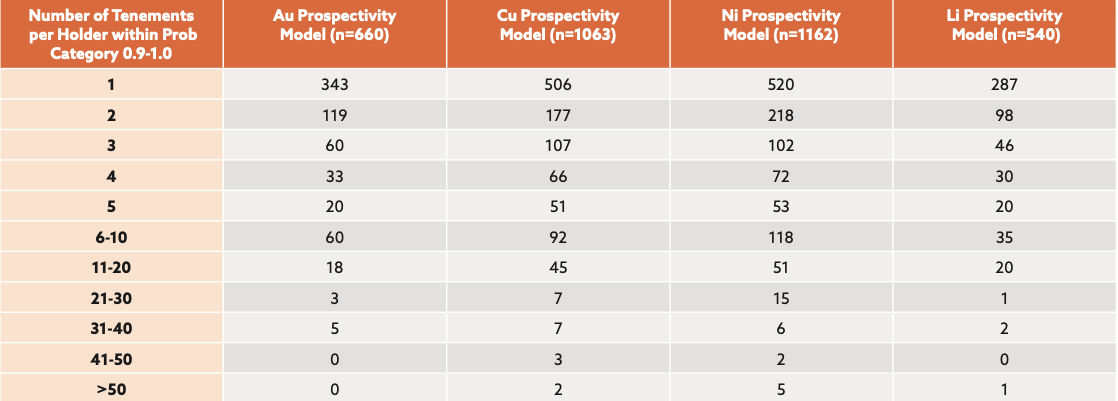

For companies that hold more than 20 of the highest-ranked tenements across the Au, Cu, Ni, and Li GPred models, we can say the following:
- For Au: 3 of the top 8 holders are producing gold, 4 are exploring for gold and the remaining 4 are not producing nor exploring for gold
- For Cu: none of the holders are producing Cu and only 1 out of 19 are exploring for Cu,
- For Ni: only 3 of the top 28 holders are producing Ni, and 2 are exploring for Ni
- For Li: none of the top 5 holders are producing nor exploring for Li
Across all four GPred models, only 29 out of the 3,497 registered tenement holders in WA hold more than 20 tenements in the highest-ranking Probability Category.
What does the 2023 Exploration Drilling Results say about our GPred models?
The GPred models were constructed by training and testing geophysics on geochemically anomalous point data for 47 elements. These models’ strength lies in using one type of data (geochemistry) to train another (geophysics) on a large scale. The probability maps produced highlight areas likely to have geochemical anomalies in regions currently lacking geochemical data, representing true predictions and new discovery opportunities.
SensOre cannot independently validate the predicted anomalies across the entire state. However, we can examine the 2023 exploration drilling results reported on the ASX and significant intersections compiled by S&P Global to see if industry-reported drilling results corroborate our modelled predictions. For gold exploration, a significant proportion (36.7%) of the most significant intersections for Au (g/tonne) reported from 1,396 WA exploration licences from Air Core drilling campaigns are on tenure that captures some of our highest probability gold anomalism predictions (GPred Au probability > 0.8) (Figure 5).
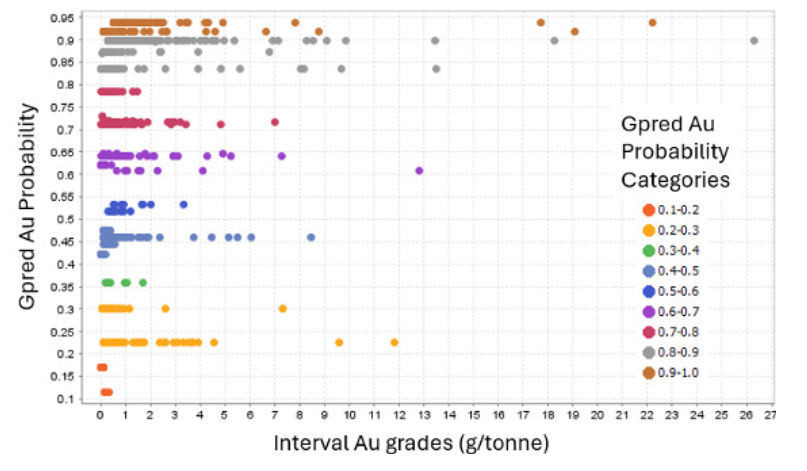

Why is SensOre’s approach to predictive modelling worth talking about?
SensOre’s Predictive Models deliver innovative insights at the resolution needed to address decision-making drivers for major mining companies and junior explorers. These insights are also valuable to investors and stakeholders in exploration and mining who seek a competitive advantage in a volatile market. Our models highlight opportunities for:
- Continued economic discovery in known mineral belts through confirmed and predicted extensions of geochemical anomalism.
- Identifying new prospective areas within metallogenic belts by highlighting previously unmapped and/or incorrectly mapped lithologies that are favourable hosts for mineralisation.
- Opening new greenfields exploration frontiers, including areas under cover that conceal new mines from conventional detection techniques.
- Facilitating rapid and intelligent area reduction by creating more discrete, higher-quality targets, reducing the time and cost required to advance a target to the next pipeline stage.
- Providing an objective data-driven metric to rank existing tenure prospectivity both within and across tenement portfolios, enabling the creation of industry-wide prospectivity league tables.
How do you get access to these resources?
Contact SensOre to maximize your exploration success by utilising our propriety advanced machine learning capabilities. SensOre is here to help you get the most out of your geological data on your way to making a discovery.